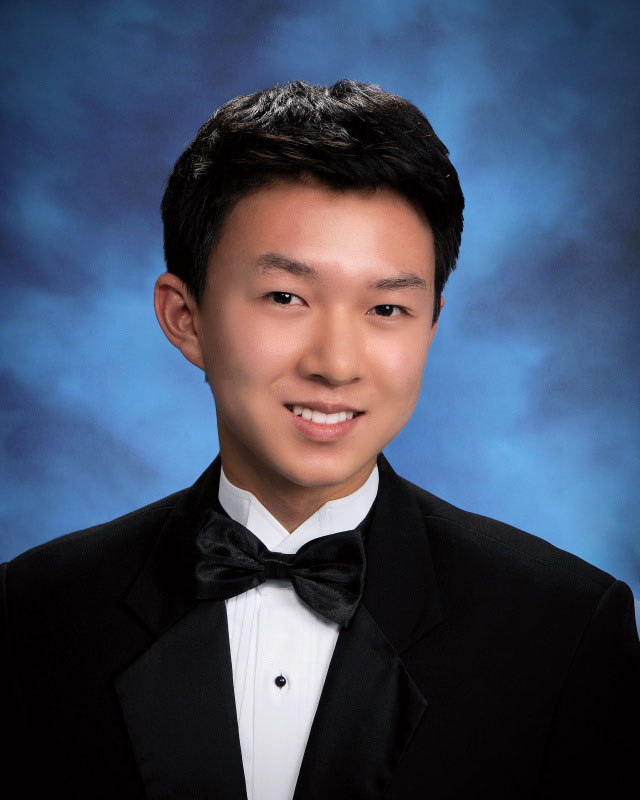
Christopher C. 2024 | BASIS Independent Fremont
- Project Title: Detecting Fast-moving Near-Earth Objects with a Novel Deep Learning Algorithm
- BASIS Independent Advisor: Dr Sanhita Dixit
- Internship Location: University of Florida
- Onsite Mentor: Dr. Jian Ge
Near-Earth Objects (NEOs) pose a significant risk to humanity. Just over 10 years ago, the Chelyabinsk meteorite collided with Earth, releasing 30 times the energy of the Hiroshima atomic bomb and injuring ~1500 people. NEOs like the Chelyabinsk meteorite are estimated to collide with Earth every 10-60 years, yet only 0.1% of these NEOs have been tracked. Major factors contributing to the low detection percentage include the time-intensive pipeline for NEO detection and current softwares’ insensitivity to faint, fast-moving NEOs. Especially fast-moving near-Earth objects that pass dangerously close to Earth leave a visible streak in telescope images. Existing research has already proven the efficacy of utilizing synthetic streak training data to train convolution neural networks (CNNs) for NEO detection. In this project, I seek to further increase the accuracy and efficiency of existing algorithms in detecting NEO streaks in images from the Zwicky Transient Facility (ZTF). I also aim to develop an efficient postprocessing pipeline to further augment the NEO identification and verification pipeline.