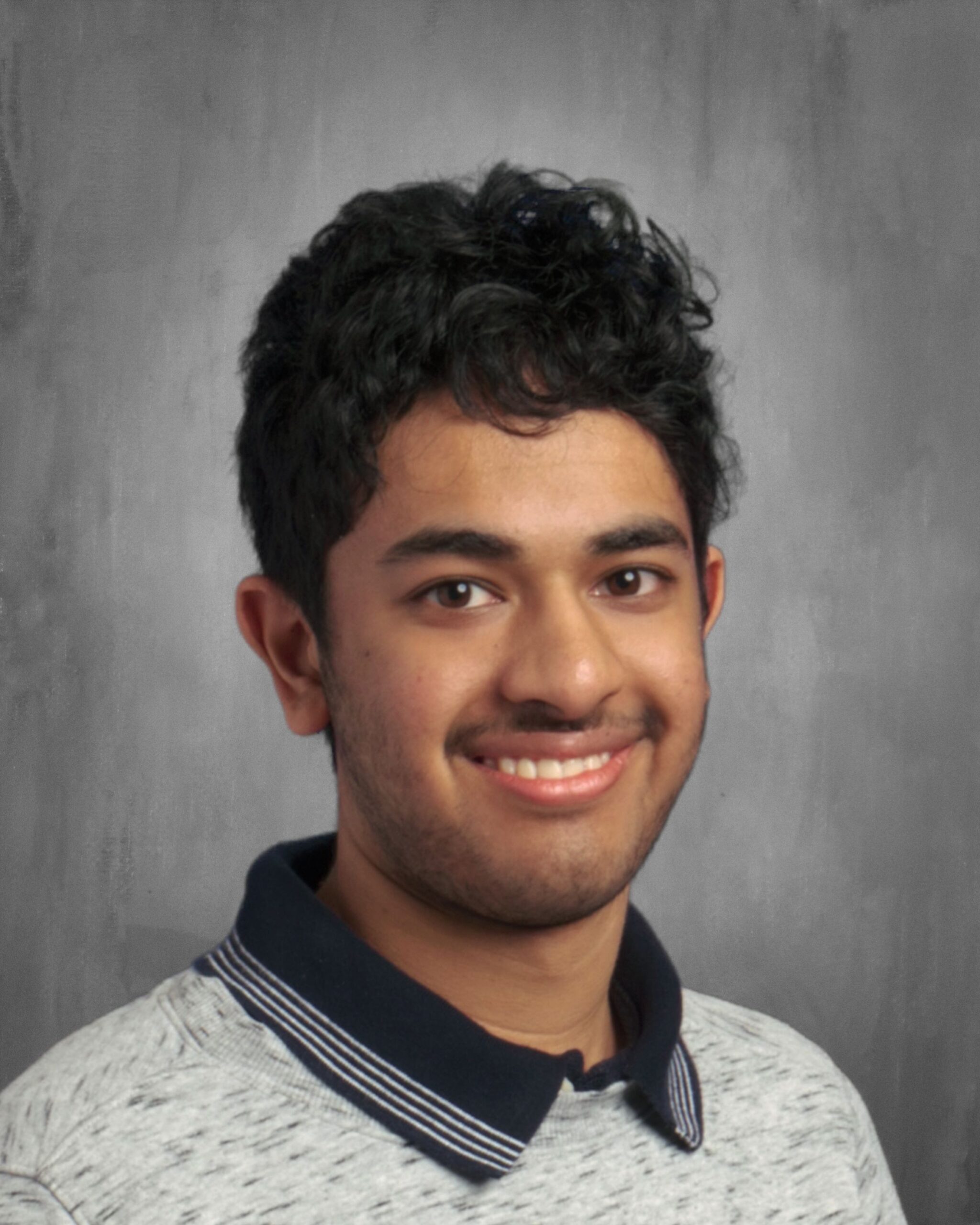
Vishak S. 2023 | BASIS Independent Silicon Valley
- Project Title: Assessing Imputation Autoencoders for Genomic Variation and Their Applications to Predictive Tasks for Precision Medicine
- BASIS Independent Advisor: Swetha Bhattacharya
- Internship Location: Scripps Research Translational Institute
- Onsite Mentor: Dr. Salvatore Loguercio
Deep learning models built from an individual’s genomic profile provide exciting new avenues for early detection or prevention of common heritable diseases like Coronary Artery Disease (CAD). For such diseases, using the observed locations of a small subset of disease-linked Single Nucleotide Polymorphisms (SNPs)—specific genomic locations where we know an individual's genome differs from the most common variant or nucleotide—we can infer unobserved genetic variation sites across the entire genome. This process, called genotype imputation, allows us to generate all known common genetic variations without incurring the costs of sequencing whole genomes of individuals. During my summer internship in Torkamani lab, I researched the use of autoencoder models, which are deep neural networks that excel at taking corrupted or masked data as input and outputting the original uncorrupted data, for genotype imputation. For my senior project, I am building an analytical framework to characterize the structure, accuracy, and efficacy of the denoising autoencoder models for genotype imputation that were developed in our lab for genetic markers of CAD. I am testing this framework on high-performance computing clusters at SRTI using specialized genomic datasets from Scripps. Specifically, I hope to discover whether the representations learned from a collection of fully trained denoising autoencoders are sufficient for individual risk prediction, the task of estimating the probability that an individual is susceptible to CAD. My research provides a key clinical decision-making tool useful in precision medicine applications for preventing or delaying the onset of CAD.